Exploring Generative AI Part 1

Generative AI has become a global phenomenon since the public release of AI chatbots such as ChatGPT however it’s not just consumers interested in exploring what generative AI has to offer. Many industries are investigating the ways generative AI can redefine existing processes and enable new, previously impractical ideas to breakdown the barriers between people, information, and technology.
In this episode, host Spencer Acain is joined by Dr. Justin Hodges, an AI/ML Technical Specialist and Product Manager for Simcenter to discuss the many applications of Generative AI to the CAE process and what that means for the future of product design.
In this episode you will learn:
- What is Generative AI? (2:56)
- Applications of Generative AI in simulation and design (7:44)
- How Generative AI eases the burden on users (9:25)
- How Generative AI makes data easier to access (11:34)
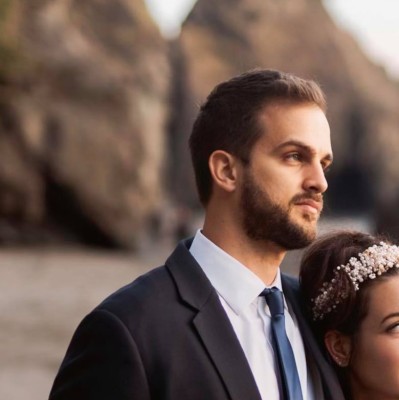
Dr. Justin Hodges
AI/ML Technical Specialist and Product Manager forSiemens Simcenter.
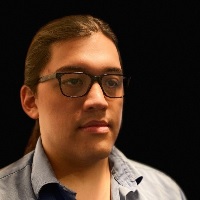
Spencer Acain
Technical Writer for Global Marketing at Siemens Digital Industries Software
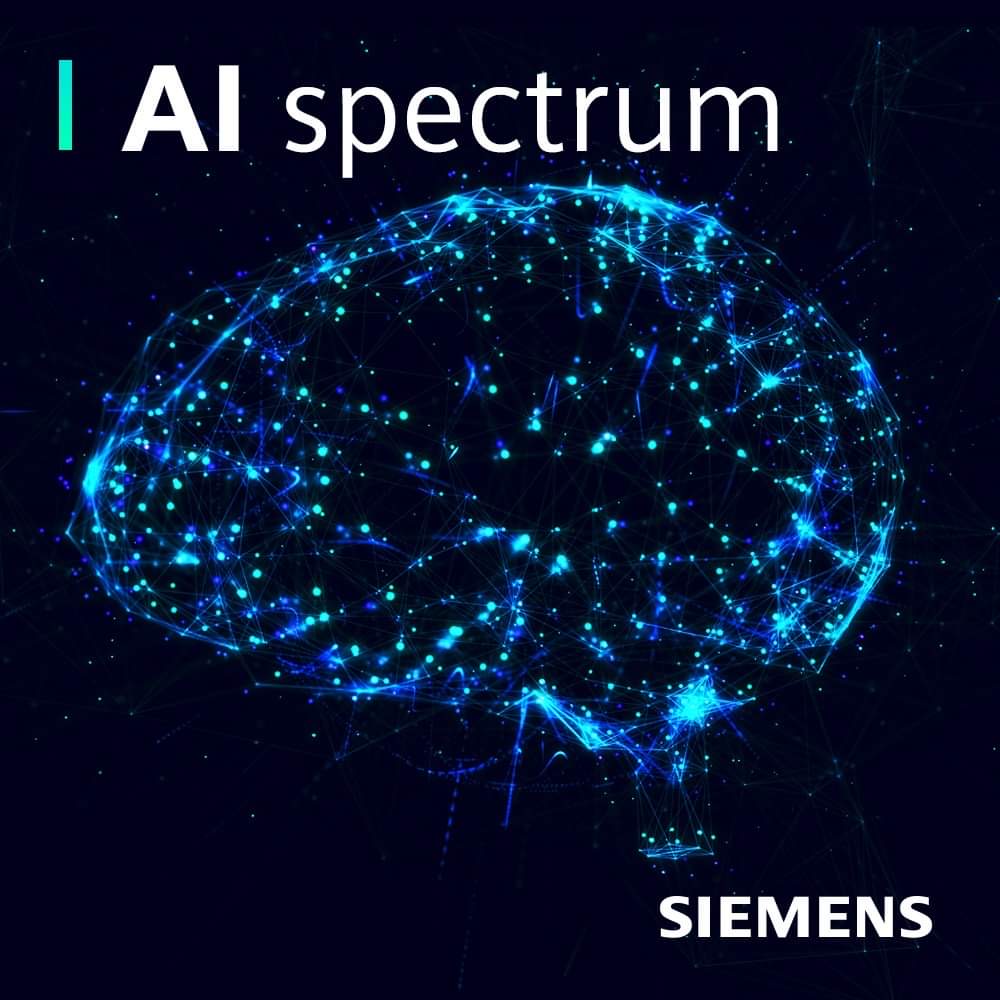
AI Spectrum
This podcast features discussions around the importance of AI and ML in today’s industrial world.
Listen on:
Comments
Leave a Reply
You must belogged into post a comment.
Generative AI is a branch of artificial intelligence focused on creating data, content, or artifacts that are not simply copied from existing sources, but rather generated from scratch based on patterns and rules learned from a given dataset. This technology has found applications in various fields such as art, music, text generation, image synthesis, and more. In this “Exploring Generative AI” series, we’ll cover the fundamental concepts, techniques, and applications of generative AI. Let’s start with Part 1:
**1. **Introduction to Generative AI**:
Generative AI refers to algorithms and models that can create new and original content that resembles certain characteristics of the training data it was exposed to. Unlike other types of AI that focus on classification or prediction, generative models aim to generate new instances of data that fit within the distribution of the training data.
* * 2。* * Types of Generative Models**:
There are various types of generative models, including:
– **Generative Adversarial Networks (GANs)**: GANs consist of two neural networks, a generator, and a discriminator, that compete against each other. The generator creates fake data, and the discriminator tries to distinguish between real and fake data. Through this competition, the generator improves over time.
– **Variational Autoencoders (VAEs)**: VAEs are encoder-decoder networks that learn a probabilistic mapping between the input data and a latent space. They generate data by sampling from the learned latent space distribution.
– **Recurrent Neural Networks (RNNs) and LSTM Networks**: These networks can generate sequences of data, such as text or music, by predicting the next item in the sequence based on the patterns it learned from the training data.
– **Transformers**: Transformers have gained popularity in natural language processing tasks and can generate text by attending to different parts of the input data.
**3. **Training Generative Models**:
Training generative models involves providing them with a large dataset of examples from the target distribution. The model learns the underlying patterns and structures of the data through optimization techniques like backpropagation and gradient descent.
**4. **Challenges and Considerations**:
Generative AI comes with its own set of challenges, such as mode collapse (in GANs), training stability, ensuring diversity in generated outputs, and ethical concerns around generating realistic but potentially harmful content.
**5. **Applications of Generative AI**:
Generative AI has a wide range of applications:
– **Image Generation**: GANs can generate realistic images, even those that never existed before.
– **Text Generation**: RNNs and transformers can generate human-like text, aiding in content creation, chatbots, and more.
– **Music Composition**: Generative models can compose new music pieces based on existing compositions.
– **Art and Design**: Generative models are used to create digital art and design elements.
– **Data Augmentation**: Generative techniques can be used to generate additional training data, improving the performance of machine learning models.
https://www.receiptify.one/
– **Drug Discovery**: Generative models can help in generating new chemical compounds for drug discovery.
In Part 2, we’ll dive deeper into some of these generative models, explore their architectures, and provide practical insights into working with them.